The Life of a Digital Agency in the Time of AI
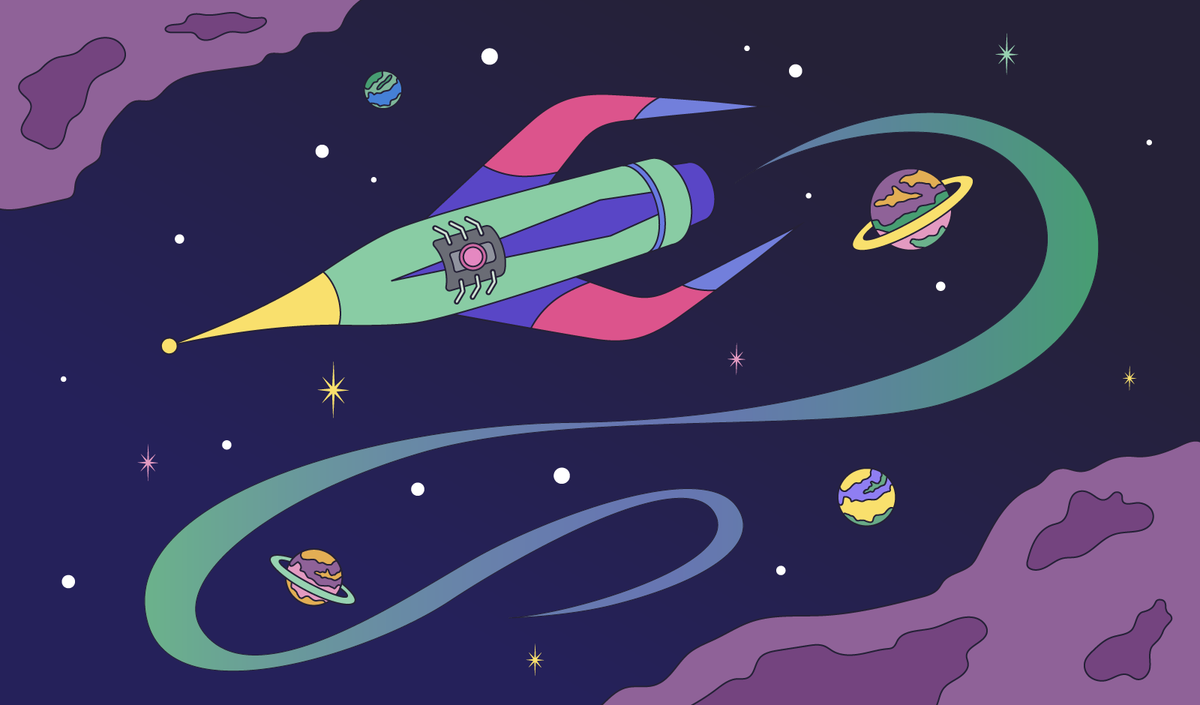
The Gartner Hype Curve
As a digital agency, we've lived through several cycles of inflated expectations — followed by the 'trough of disillusionment,' the 'slope of enlightenment,' and the eventual 'plateau of productivity'.
Gartner hype curve. Copyright © Wikipedia .
It Should All Be Free as in Beer
The first notable cycle — followed by gradual enlightenment and productivity — was the expectation that all things digital or online should somehow be cheap, or even free.
There are a few reasons why companies in the early days of the web (and again today in the age of AI) expected digital products to be cheap or even free:
- Perceived Intangibility = Perceived Low Cost: Unlike print — where there’s paper, ink, presses, binding, and freight — digital products are effectively virtual. If something doesn’t have a physical cost, many assume it shouldn’t cost much at all. A web page loads instantly. There's no sense of material or manual labor — just code that “appears.” The effort behind that code — design, development, UX, QA, content — is invisible.
- Commodification of Creative Work: Digital design and development, unlike high-end print or broadcast production, quickly became commodified. Once templates and off-the-shelf themes appeared, companies stopped comparing digital work to a bespoke design process and started comparing it to $29 downloads. The nuanced thinking, research, and problem-solving behind a well-made digital experience became hard to see.
- Confusion Between Output and Outcome: Many organizations confused having a website with having a good website. The bar for quality was unclear. To them, success wasn’t necessarily tied to user experience, accessibility, performance, or a return on their investment — it was about "being online." If the output could be produced quickly and looked “okay,” then that was seen as good enough.
- The Myth of Scale Without Cost: The internet promised infinite reach. A digital property could potentially reach millions overnight. But with that came the mistaken idea that scaling online was cheap — and therefore creating something to scale should be cheap too. They missed that the infrastructure and expertise required to scale, still takes craft, effort and resources, no matter how far a product might travel.
Over time, organizations of all sizes began to realize that being online didn’t eliminate the need for careful planning and a multidisciplinary approach when building quality digital products.
It's All in the Cloud
The second notable cycle — the myth of “The Cloud will solve everything” — was (and still is) a huge shift in expectations (and an evolution of "The Myth of Scale Without Cost"), especially for smaller agencies trying to set honest boundaries with clients.
Here's why so many people fell into that belief:
- “The Cloud” Was Marketed as Magic: Cloud services were pitched with phrases like "No more servers," "Zero maintenance," and "Instant scalability." That marketing made it sound like everything — hosting, backups, security, monitoring, patching — would be automatically handled. What got missed in translation was that someone still has to architect, configure, monitor, and support all those pieces.
- Misunderstanding of What ‘Cloud’ Actually Means: For many clients, "the cloud" was just a vague concept — not a shift in infrastructure models, but a sort of digital heaven where problems dissolve. They didn’t realize that AWS, GCP, or Azure are not turnkey platforms — they're toolkits. They're like giving someone all the pieces of a spaceship and saying, "You can launch now!" without explaining the complexity.
- “Set and Forget” Wishful Thinking: Clients often saw cloud hosting as a way to stop thinking about infrastructure. Once it was “in the cloud,” it was someone else’s problem. That mindset is dangerous — because cloud environments still require configuration, budgeting, security hardening, scaling logic, and regular upkeep. The costs and risks are still real, even if they've moved behind an API or a dashboard.
- Pricing Models Hid the Real Costs: The pay-as-you-go pricing model made it look like cloud was cheaper and simpler. But unless things are architected carefully, cloud bills can spike fast — and troubleshooting cloud performance issues can be just as hard, if not harder, than dealing with traditional infrastructure. Clients didn’t always grasp that ease of provisioning doesn’t necessarily translate into reduced total cost of ownership.
Just like in the first wave — when people thought websites were cheap because they were digital — the cloud created a similar illusion: if it’s in the cloud, then it must be fast, cheap, and effortless. This isn't anecdotal waffling. We've sat in meetings with senior decision makers for well-known and large organizations, where operational concerns were dismissed with statements like - "oh, it will all be in the cloud".
Only recently are we seeing a true slope of enlightenment related to cloud computing, with some services shifting back to mixed or more traditional infrastructure, combined with more careful planning around contingency costs that have caught many organizations by surprise.
AI Can Do Anything You Can Do
And so here we are in our third wave of inflated expectations — the AI wave — and while different in scale, it's eerily familiar in shape.
Clients (and sometimes even agencies themselves) are being swept up in the belief that generative AI can do everything: design, write, code, think, plan, innovate — all instantly, all correctly, and all without cost.
But that belief rests on a profound misunderstanding of what generative AI and large language models (LLMs) actually are — and aren’t.
To begin with, LLMs don’t actually know anything. They don’t plan. They don’t reason. They don’t form intent or understand purpose. They’re incredibly sophisticated autocomplete engines — guessing the most likely next word or pixel based on patterns in the data they were trained on.
What they produce is an echo — a remix of other people’s work, other people’s insights. And while that’s enormously useful in the right context — for brainstorming, summarizing, scaffolding — it breaks down the moment you ask LLM-based AI to do what good strategists, designers, developers, and writers do:
- Navigate ambiguity
- Think in systems
- Consider edge cases
- Predict unintended consequences
- Craft something truly novel, or appropriate to a specific real-world context
AI can give you a plan — but not your plan.
It can give you a brand voice — but not your voice.
It can write code — but not necessarily the code you want.
So as an agency, we now face another round in managing client expectations: Explaining not just what AI can do, but what it can’t do — and why the real value of a digital product still lies in human expertise, collaboration, and judgment.
Perhaps even more ironic is that knowing whether AI is good or bad at something requires having the skills and experience to know the difference. And therefore the real risk, is that as time goes on, fewer people will have these skills as judgment earned through experience is weakened by the misapplication of AI.
When we finally reach the slope of enlightenment and the plateau of productivity in the AI wave, things will look very different. There’s no denying it’s going to have a major impact on what our clients expect, and how we work.
Until that time — hang on for the ride, because this wave is going to sweep away the coastline.